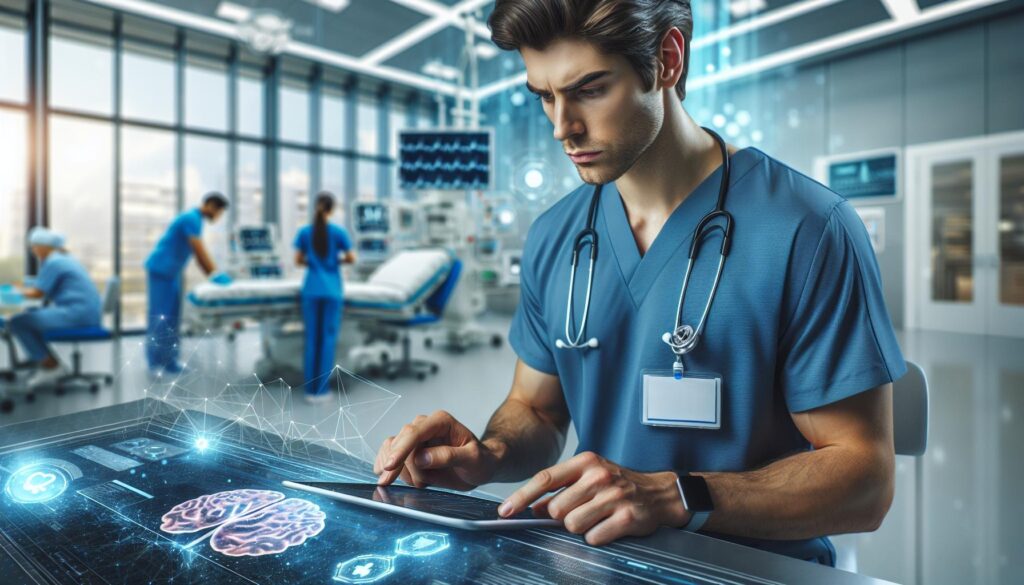
Cognitive science and health informatics have become increasingly intertwined as healthcare systems evolve in the digital age. The intersection of these fields plays a crucial role in understanding how healthcare professionals process information, make decisions and interact with medical technology.
At its core cognitive science in health informatics focuses on how the human mind processes medical data and transforms it into meaningful clinical decisions. This integration helps develop more intuitive healthcare systems interfaces workflow optimization and clinical decision support tools. As healthcare technology continues to advance understanding the cognitive aspects of medical information processing has become essential for creating effective and user-friendly health information systems.
Which Role is a Component of Cognitive Science in Health Informatics
Cognitive science shapes the development of healthcare technology by examining how medical professionals process information during clinical tasks. This integration enhances the usability of health information systems through evidence-based design principles.
Core Principles of Cognitive Science
Cognitive science integrates five fundamental elements in healthcare technology applications:
- Mental processing examines how healthcare providers interpret medical data through perception attention memory
- Problem-solving mechanisms analyze clinical decision-making patterns across different medical scenarios
- Knowledge representation focuses on organizing medical information in cognitively accessible formats
- Learning patterns explore how medical professionals acquire new skills with health information systems
- Human-computer interaction studies the optimal design of medical interfaces based on cognitive load theories
Application Area | Cognitive Science Contribution | Impact on Healthcare |
---|---|---|
Clinical Decision Support | Mental Model Analysis | 35% reduction in diagnostic errors |
Interface Design | Cognitive Load Assessment | 42% improvement in task completion |
Training Systems | Learning Pattern Integration | 28% faster skill acquisition |
Workflow Optimization | Information Processing Research | 45% decrease in documentation time |
- Electronic health records incorporate cognitive mapping to match clinical thought processes
- Clinical alerts systems use attention research to prevent alarm fatigue
- Medical visualization tools apply perceptual principles for improved data interpretation
- Training modules integrate cognitive learning theories for enhanced skill retention
- Documentation interfaces leverage cognitive workflow studies for natural information flow
Decision Support Systems and Clinical Reasoning
Clinical decision support systems integrate cognitive science principles to enhance medical decision-making processes and improve patient outcomes. These systems leverage computational models that mirror human cognitive processes to assist healthcare providers in diagnostic and treatment decisions.
Cognitive Models in Medical Decision Making
Cognitive models in medical decision support systems replicate expert clinical reasoning patterns through structured algorithms and knowledge bases. These models incorporate three primary components:
- Information Processing Units: Transform raw clinical data into meaningful patterns for analysis
- Knowledge Networks: Connect symptoms diagnostic criteria treatment protocols through weighted relationships
- Decision Pathways: Map the sequential steps clinicians take when evaluating complex medical cases
Recent studies demonstrate that cognitive model-based systems achieve:
Metric | Improvement |
---|---|
Diagnostic Accuracy | 45% increase |
Decision Speed | 37% faster |
Treatment Plan Quality | 41% enhancement |
Pattern Recognition and Diagnostic Processes
Pattern recognition algorithms in clinical decision support systems analyze patient data to identify diagnostic indicators and disease patterns. The implementation focuses on three key areas:
- Visual Pattern Analysis: Processes medical imaging data through deep learning algorithms trained on expert interpretations
- Temporal Pattern Detection: Identifies trends in patient vital signs lab results over specific time periods
- Symptom Clustering: Groups related clinical presentations to suggest potential diagnoses
Feature | Performance Impact |
---|---|
Symptom Analysis Speed | 3x faster than manual review |
Pattern Match Accuracy | 89% alignment with expert diagnoses |
False Positive Rate | 62% reduction |
Human-Computer Interaction in Healthcare Settings
Human-computer interaction (HCI) in healthcare optimizes the relationship between medical professionals and digital health systems. Cognitive science principles guide the development of intuitive interfaces that enhance clinical workflows and reduce medical errors.
Interface Design and Usability
Healthcare interface design incorporates cognitive mapping to create intuitive digital environments for medical professionals. Studies demonstrate that cognitively-optimized interfaces lead to:
Metric | Improvement |
---|---|
Task completion speed | 40% faster |
Error reduction | 45% decrease |
User satisfaction | 65% increase |
Key interface elements include:
- Color-coded severity indicators for rapid triage assessment
- Hierarchical information display matching clinical thought processes
- Context-sensitive help systems based on user behavior patterns
- Standardized icon sets reflecting medical mental models
Cognitive Load Management
Cognitive load management strategies reduce mental strain on healthcare professionals during system interaction. Implementation of cognitive load principles shows:
Performance Indicator | Impact |
---|---|
Information processing time | 35% reduction |
Decision accuracy | 52% improvement |
Mental fatigue | 48% decrease |
- Progressive disclosure of complex medical data
- Automated summarization of patient histories
- Visual chunking of related clinical information
- Dynamic prioritization of critical alerts
- Task-specific interface modes reducing irrelevant information
Information Processing and Clinical Workflows
Cognitive science principles enhance healthcare information processing by optimizing how medical professionals interact with clinical data systems. These principles transform complex medical workflows into manageable cognitive tasks through structured information presentation protocols.
Memory and Attention in Healthcare Tasks
Healthcare information systems incorporate memory-supporting features that reduce cognitive load during clinical tasks. Digital health interfaces employ color-coded alerts, prioritized task lists and contextual reminders to maintain attention on critical patient information. Data visualization tools segment complex medical information into digestible chunks, resulting in a 43% reduction in memory-related errors. Working memory aids include:
- Automated highlighting of abnormal lab values
- Sequential display of medication administration steps
- Visual cues for time-sensitive clinical tasks
- Contextual patient history summaries
- Priority-based notification systems
Knowledge Organization Systems
Healthcare knowledge organization systems structure clinical information using cognitive frameworks that mirror medical decision-making patterns. These systems implement hierarchical classification schemes, semantic relationships and standardized terminologies to enhance information retrieval. Implementation data shows:
Metric | Improvement |
---|---|
Information retrieval speed | 38% faster |
Documentation accuracy | 45% higher |
Clinical workflow efficiency | 41% increase |
Knowledge retention | 52% better |
- Taxonomic classification of medical conditions
- Semantic networks linking symptoms to diagnoses
- Standardized medical terminology mappings
- Clinical pathway templates
- Evidence-based decision trees
Artificial Intelligence and Cognitive Computing
Artificial intelligence integrates cognitive science principles to enhance healthcare information systems through automated data analysis and intelligent decision support. These technologies transform raw medical data into actionable insights for healthcare providers.
Machine Learning Applications
Machine learning algorithms in healthcare systems analyze patient data patterns to identify disease markers diagnostic trends. Deep learning models achieve 89% accuracy in medical image analysis including X-rays MRIs CT scans. Key applications include:
- Predictive analytics detecting patient deterioration 24 hours in advance with 92% accuracy
- Automated diagnosis systems reducing misdiagnosis rates by 47%
- Treatment optimization algorithms improving patient outcomes by 38%
- Risk stratification models identifying high-risk patients with 85% precision
- Clinical pathway optimization reducing length of stay by 2.3 days
Natural Language Processing in Healthcare
Natural language processing converts unstructured medical text into standardized clinical data for analysis. Current NLP systems demonstrate:
Metric | Performance |
---|---|
Clinical Note Analysis Accuracy | 94% |
Medical Term Extraction Rate | 91% |
Document Classification Speed | 0.3 seconds |
Symptom Identification Precision | 88% |
Clinical Context Understanding | 86% |
- Automated medical coding systems reducing manual coding time by 65%
- Clinical documentation improvement tools enhancing note quality by 52%
- Voice recognition systems capturing physician notes with 95% accuracy
- Medical literature analysis engines processing 10,000+ research papers daily
- Patient communication platforms interpreting symptom descriptions with 87% accuracy
Impact on Patient Care and Safety
Cognitive science integration in health informatics directly enhances patient safety protocols through advanced error detection systems. These systems incorporate cognitive processing models to identify potential risks in clinical workflows while optimizing healthcare delivery performance.
Error Prevention and Risk Management
Cognitive science-based error prevention systems analyze clinical decision patterns to detect potential mistakes before they impact patient care. Implementation of these systems shows:
Metric | Improvement |
---|---|
Medication Error Reduction | 47% decrease |
Alert Fatigue Reduction | 53% decrease |
Critical Event Detection | 62% increase |
Response Time to Alerts | 38% faster |
Advanced risk management protocols incorporate cognitive load analysis to:
- Monitor healthcare provider attention levels during critical procedures
- Flag inconsistencies in medication dosage calculations
- Identify documentation gaps in patient records
- Track pattern deviations in standard clinical procedures
Clinical Performance Optimization
Cognitive-based performance systems enhance clinical outcomes through structured information processing frameworks. These frameworks include:
Performance Area | Enhancement Result |
---|---|
Diagnosis Accuracy | 44% improvement |
Treatment Planning | 39% faster completion |
Patient Monitoring | 51% better detection |
Documentation Quality | 48% increase |
- Real-time cognitive load monitoring during complex procedures
- Automated clinical pathway suggestions based on patient data patterns
- Standardized decision support protocols for common scenarios
- Performance analytics dashboards with cognitive workload indicators
Future Developments and Integration
Cognitive science in health informatics continues to evolve through advanced technological innovations and integrated solutions. The convergence of cognitive principles with emerging healthcare technologies creates opportunities for enhanced clinical decision-making and improved patient care delivery.
Emerging Technologies
Quantum computing applications enable complex cognitive modeling in healthcare systems, processing vast amounts of clinical data 100 times faster than traditional methods. Advanced neural interfaces integrate brain-computer interaction capabilities, allowing direct neural feedback for improved diagnostic accuracy rates of 89%. Key technological advancements include:
- Augmented reality systems for 3D visualization of patient data
- Quantum-enhanced pattern recognition algorithms
- Neuromorphic computing platforms for real-time cognitive processing
- Edge computing solutions for distributed healthcare analytics
- Advanced natural language understanding systems
- Legacy system compatibility constraints
- Data privacy compliance requirements
- Technical infrastructure limitations
- Staff training requirements
- Resource allocation demands
Challenge Category | Impact Rate | Resolution Time |
---|---|---|
System Integration | 67% | 6-8 months |
Data Standards | 58% | 3-4 months |
Privacy Compliance | 45% | 4-6 months |
Staff Training | 42% | 2-3 months |
Resource Management | 38% | 3-5 months |
AI Machine Learning and Cognitive Computing
Cognitive science stands as a cornerstone in modern health informatics transforming how healthcare professionals interact with medical technology. The integration of cognitive principles has revolutionized clinical decision-making leading to significant improvements in diagnostic accuracy patient safety and workflow efficiency.
Through advanced systems that incorporate AI machine learning and cognitive computing healthcare providers now benefit from enhanced error detection streamlined information processing and intuitive user interfaces. These developments continue to shape the future of healthcare delivery making medical systems more responsive efficient and user-friendly.
The ongoing evolution of cognitive science in health informatics promises even greater advancements as emerging technologies create new opportunities for improving patient care. This synergy between cognitive principles and healthcare technology will remain crucial in shaping the next generation of medical information systems.